Decoding The AI Black Box In Drug Research : A Revelation in Machine Learning
- Blogo Tomato
- Feb 9, 2024
- 2 min read
Updated: Feb 15, 2024
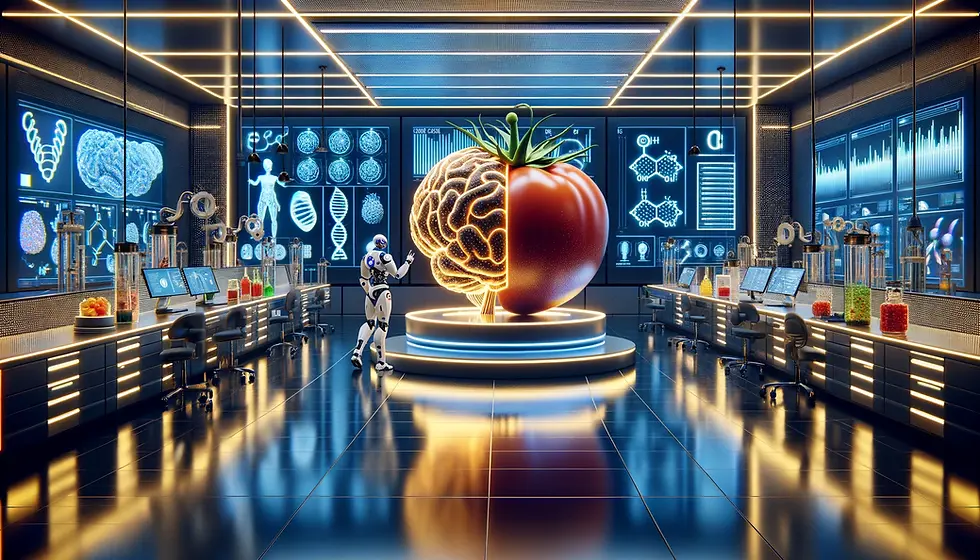
Welcome back, dear reader! Today's post takes us on an insightful journey into the ever-changing world of AI in drug research; brought to you by a recent study by colleagues at the University of Bonn and Sapienza University in Rome has brought to light some surprising findings about Graph Neural Networks (GNNs) as an exciting, novel staple in pharmaceutical research.
For years, AI's role in drug discovery has been likened to something akin to a “black box” - mysterious and often impenetrable. But this study, led by Prof. Dr. Jürgen Bajorath and Andrea Mastropietro, unveils the inner workings of these GNNs, providing us with a clearer picture of their capabilities and limitations.
The study focused on how GNNs, trained with graphs representing protein-ligand complexes, predict the binding strength of molecules to target proteins. Using a method called EdgeSHAPer, the researchers discovered that instead of learning specific chemical interactions, the GNNs were largely 'remembering' chemically similar molecules encountered during their training. This phenomenon mirrors the "Clever Hans effect," where predictions are made based on learned similarities rather than a deep understanding of chemical interactions.
These findings challenge the notion that GNNs are learning chemical interactions between substances and proteins. Instead, their predictions are heavily reliant on the data they've been trained with, highlighting a need for more sophisticated training techniques and models.
But it's not all a tale of limitations. The study also revealed that some GNN models showed a tendency to learn more interactions with increased potency of test compounds. This opens a window for further improvement and fine-tuning of these AI models in drug discovery!
As we navigate the twists and turns of AI in drug research, studies like this are vital. They shed light on the so-called mysterious black box of AI, guiding us toward more effective and transparent AI applications. And in Tomatopia, where innovation and clarity are key, this study represents a step forward in our understanding of AI's role in revolutionizing drug discovery.
Stay tuned to Tomato Blogs for more exciting updates from the AI world, where technology meets transparency and innovation blooms!
All Hail The Mother Tomato.
Comments